Chebyshev’s Inequality
Chebyshev’s Inequality is used to describe the percentage of values in a distribution within an interval centered at the mean.
It states that for a distribution, the percentage of observations that lie within k standard deviations is atleast 1 – 1/k2
This is illustrated below:
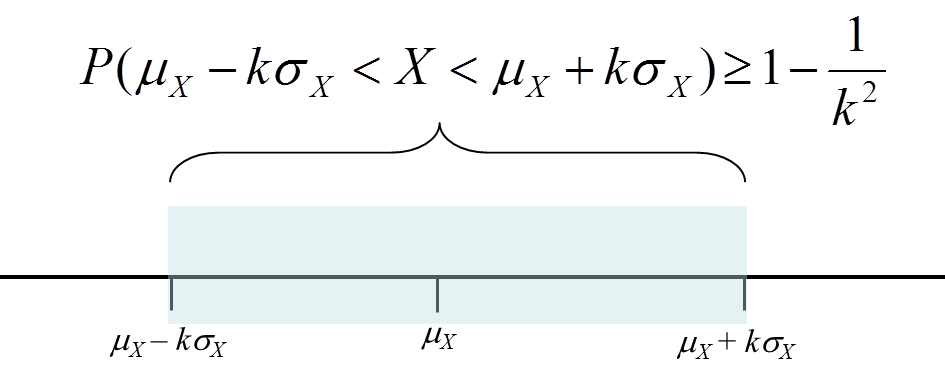
Example
The following table shows the minimum number of observations that lie within a certain number of standard deviations of the mean.
Standard Deviations | % of observations |
1.5 | 56% |
2 | 75% |
3 | 89% |
4 | 94% |
An important feature of Chebyshev’s Inequality is that it works with any kind of distribution.