What is Machine Learning?
Machine Learning is the field which applies statistical analysis and computer science for employing algorithms that learn how to perform tasks such as prediction or classification of a target variable as well as grouping data. These algorithms learn from data and are widely diverse as they range from traditional statistical models based on inference to complex deep neural networks architectures.
Machine learning has many applications in quantitative finance and their techniques have evolved and improved very fast in recent years.
Some examples of problems that could be addressed with machine learning are the following:
- Prediction of future asset price movements
- Prediction of liquidity movements due to redemption of capital in large funds
- Finding mispricing opportunities in niche markets
- Categorize stocks for optimizing portfolio allocations
- Image recognition for use in commodity supply/demand signals
- Natural Language Processing to gauge market sentiment and create trading signals for asset price forecasting
The big three categories in the machine learning field are Supervised Learning, Unsupervised Learning and Reinforced Learning. All three methods have their own procedures and are used for different tasks such as prediction, find data patterns, categorizing data among others.
In this tutorial, we will focus on Supervised and Unsupervised Learning and we will provide an explanation of both fields showing examples in python programming for a practical overview and giving theoretical explanation to understand how these models works.
Machine Learning Steps
Working in machine learning involves many skills not only from statistics and mathematics, but also in the field of data cleaning, and data preparation/preprocessing. Along this tutorial, we will focus in data preprocessing, training and testing models, model selection, performance evaluation and hyper-parameter tuning (Change default values of parameters of the models). The end-to-end workflow in machine learning can be described by the following picture:
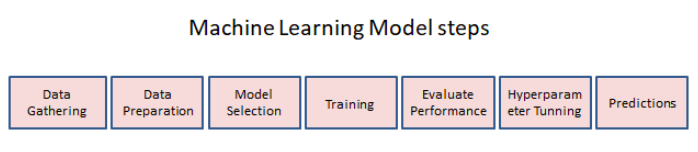
These steps at first follow this order but it is very common that once we have the predictions of the model, we start to work again on it and re-process the data in order to make new features, remove non-significant features, modify model parameters (hyperparameter tuning) and change the algorithm of the model (model selection).
In the next section we will explain important steps on the first stages of a machine learning project. Along the course we provide code examples using Scikit Learn library from Python that has already implemented a great number of machine learning algorithms.
The Scikit Learn is the most used library for Machine Learning in Python which has an extensive API for running machine learning algorithms.