How Insurance Companies Calculate Risk
People who are good at calculating probability and risk are few and far between. That is why understanding statistics is so vital to the market.
Like finance, the insurance industry is a collective of individuals who understand how risks change over time. The majority of Americans, on the other hand, find the sector overwhelming and annoying.
This article will cover the primary factors that insurance companies take into their calculation of risk.
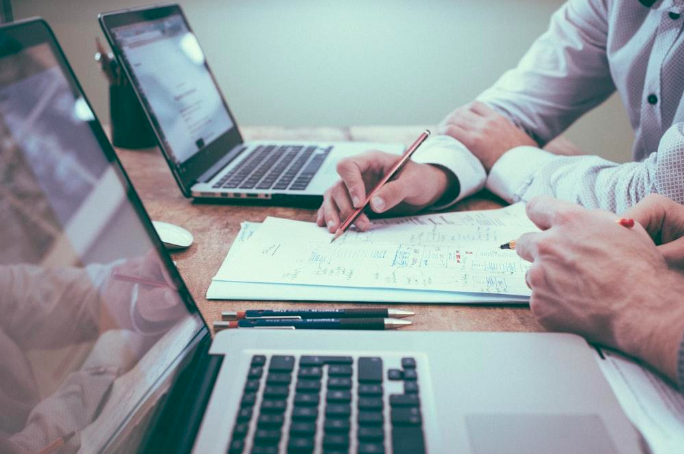
Count the Possible Catastrophes
Insurance companies take account of all of the possible terrifying and unlucky events that could happen. When calculating premiums, insurance companies make sure that they are high enough to cover the costs of damages while reaping a healthy profit. To ensure success, the industry leverages catastrophe modelling as a sub-industry.
Catastrophe Modeling uses complex computer-generated models to predict the likelihood of disasters (natural and human-made) and how much these events would cost to repair. While also determining how much money the company must collect to cover themselves.
For example, a home insurance company is calculating hurricane risks to determine the premium for a beachfront home. They will plug data about past hurricane behaviour into a model. The simulation is run about 10,000 times to provide 10,000 possible outcomes; some of these outcomes are benign; others are disastrous.
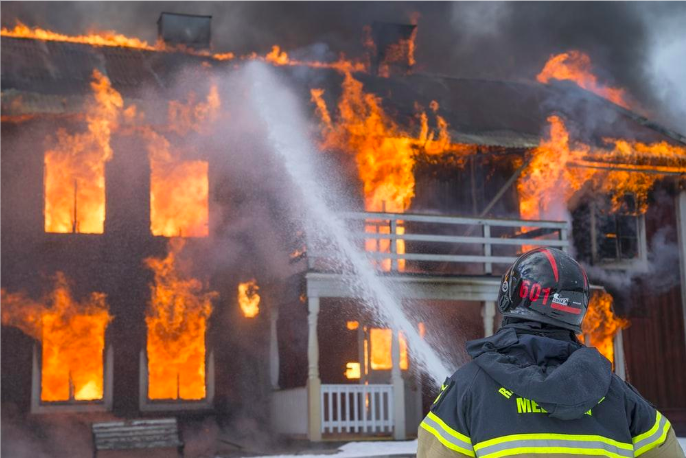
How Do Home Insurance Companies Calculate Risk?
The value of your home and its contents are not the only factors that influence how much you will have to pay each year.
Home Insurance premiums vary depending on region and insuring agency. Each home insurance company has its own criteria for determining rates. Companies look at the potential ‘risk.’
The likelihood that a homeowner will file a claim is determined mainly by the region. Weather-related claims are typically the driving force behind higher rates. As we touched on earlier, home insurance companies will run various risk models to identify the most expensive and most common culprit behind claims.
Accessibility to resources and labor also can impact the premium rate. If the home is far from fire stations, the coastline, a high crime area, or a region prone to wildfires, prices are much higher because of the risk.
The condition of a home also is considered by home insurance companies. If the property is in poor shape, the likelihood that the company will have to pay out more in a case of damage is higher, and the risk of loss is as well.
How Do Auto Insurance Calculate Risk?
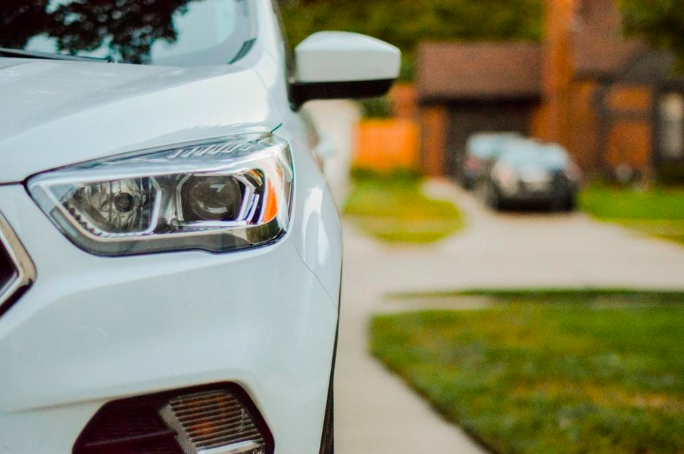
Car insurance companies take into consideration hundreds, maybe thousands of factors to determine your risk as a policyholder. Below we will list a few of the ways that auto insurance companies assess your risk as a client.
- The first thing that car insurance companies will consider is your driving record. If you are particularly accident-prone, your risk level is much higher, and so are your premiums.
- Your commute is another significant indicator. How long do you drive per day? The more you are in the car, the more risk there is of an incident.
- One primary determinant is the vehicle that you drive. If you drive a superfast sports car that is expensive to repair, you will pay more in premiums than a family with a minivan, because you are at higher risk.
- Your age matters. Many studies show those under 25 and over 65 are more likely to be in accidents.
- Insurance companies will use your credit score to determine the financial risks that you present to them as a client.
Some companies will also use marital status as an indicator. Because if you are more likely to have someone you love in the car, your automobile risks are lower. Though that may seem like a stretch, studies show that married drivers are less likely to get in car accidents.
In Summary
Calculating risk is a central task of any insurance company. The way that profit is made in the insurance industry is determined on if these projections are accurate, if not overshot. There are many factors taken into consideration when determining the premium rate for your auto and home insurance. No matter if we like it or not, at some point, we are all involved in these complex risk models.