Data Cleaning in R - Part 3
Default by States
We take a look at default rate for each state. We filter out states that have too small number of loans(less than 1000):
1tmp = data_train %>% filter(loan_status=="Default") %>% group_by(addr_state) %>% summarise(default_count = n())
2tmp2 = data_train %>% group_by(addr_state) %>% summarise(count = n())
3tmp3 = tmp2 %>% left_join(tmp) %>% mutate(default_rate = default_count/count)
4
1> tmp3
2# A tibble: 50 x 4
3 addr_state count default_count default_rate
4
5 1 AK 112 42 0.375
6 2 AL 557 194 0.348
7 3 AR 315 122 0.387
8 4 AZ 1090 316 0.290
9 5 CA 6173 1837 0.298
10 6 CO 952 222 0.233
11 7 CT 563 147 0.261
12 8 DC 86 14 0.163
13 9 DE 118 31 0.263
1410 FL 3014 933 0.310
15# ... with 40 more rows
16>
17
Order States by Default Rate
We can order states by default rate to identify states with highest and lowest default rates.
1#order by highest default rate
2
3high_default = (tmp3 %>% filter(count > 1000) %>% arrange(desc(default_rate)))[1:10,"addr_state"]$addr_state
4
5high_default
6 [1] "NY" "PA" "NJ" "OH" "FL" "IL" "NC" "MI" "TX" "CA"
7
1# order by lowest default rate
2
3low_default = (tmp3 %>% filter(count > 1000) %>% arrange((default_rate)))[1:10,"addr_state"]$addr_state
4
5> low_default
6 [1] "CO" "GA" "VA" "AZ" "CA" "TX" "MI" "NC" "IL" "FL"
7>
8
We then create binary variable for 5 highest states and 5 lowest states discard the rest.
1data_train$is_ny = ifelse(data_train$addr_state=="NY",1,0)
2data_train$is_pa = ifelse(data_train$addr_state=="PA",1,0)
3data_train$is_nj = ifelse(data_train$addr_state=="NJ",1,0)
4data_train$is_oh = ifelse(data_train$addr_state=="OH",1,0)
5data_train$is_fl = ifelse(data_train$addr_state=="FL",1,0)
6
7data_train$is_co = ifelse(data_train$addr_state=="CO",1,0)
8data_train$is_ga = ifelse(data_train$addr_state=="GA",1,0)
9data_train$is_va = ifelse(data_train$addr_state=="VA",1,0)
10data_train$is_az = ifelse(data_train$addr_state=="AZ",1,0)
11data_train$is_ca = ifelse(data_train$addr_state=="CA",1,0)
12
data_train$addr_state = NULL
rm(tmp, tmp2, tmp3, high_default, low_default)
Remove Correlated Features
We will investigate if there are any correlation among features.
1corrplot(cor(data_train[getNumericColumns(data_train)],use="na.or.complete”))
2
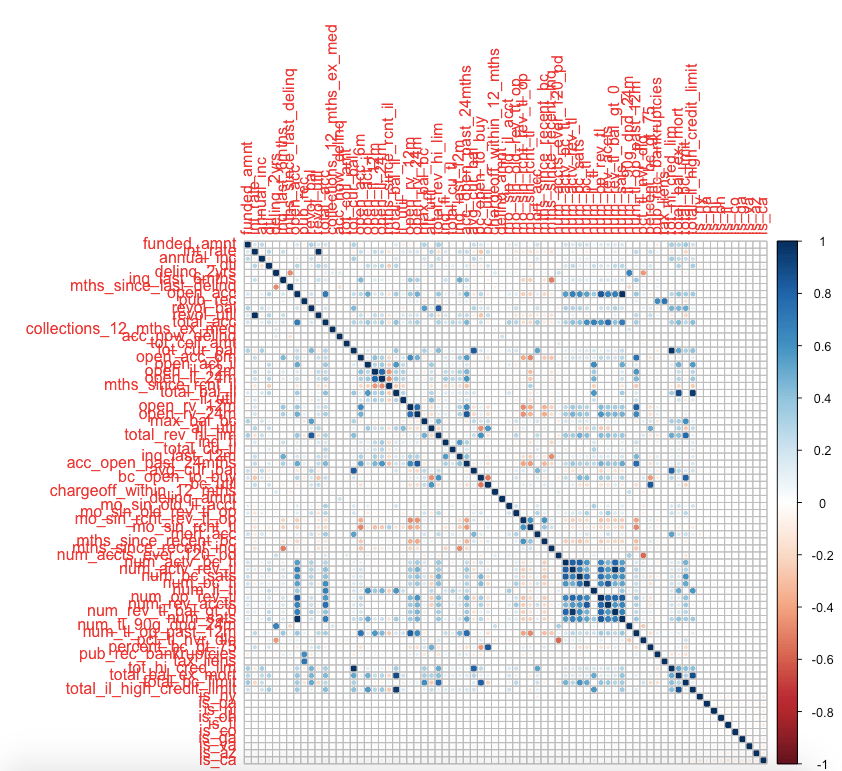
We found some features are quite correlated, we can remove correlated features with findCorrelation function. The function will find all correlated pairs that have correlation exceed a specified threshold and try to remove one of them in such a way that overall correlation is reduced.
1> high_corr <- findCorrelation(cor(data_train[getNumericColumns(data_train)]), cutoff = .75)
2> high_corr = getNumericColumns(data_train)[high_corr]
3> high_corr
4 [1] "open_acc" "num_sats" "total_acc"
5 [4] "acc_open_past_24mths" "num_op_rev_tl" "num_rev_accts"
6 [7] "num_bc_sats" "num_tl_op_past_12m" "total_rev_hi_lim"
7[10] "total_bc_limit" "total_bal_ex_mort" "num_actv_rev_tl"
8[13] "num_rev_tl_bal_gt_0" "open_rv_24m" "tot_hi_cred_lim"
9[16] "tot_cur_bal" "total_il_high_credit_limit" "open_il_24m"
10[19] "int_rate" "mo_sin_old_rev_tl_op" "bc_util"
11>
12
1data_train = (data_train[,!(names(data_train) %in% high_corr)])
2