Role of Data Science in Risk Management
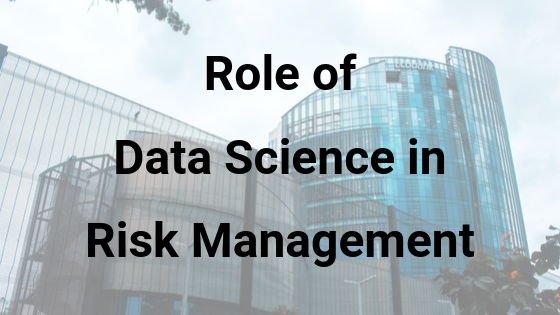
Risk management is an integral part of any financial institution. All businesses face a variety of risks and the risk management practice works towards maximizing the businesses’ return on investment and reducing their losses. Anyone who works in risk management is not new to data analytics. The field of risk management is highly dependent on data and risk professionals have been using analytics for many years. In fact, financial institutions are the hub of data and have been pioneers of data analytics. However, as we have seen, the data has exploded in the last decade and big data technologies have emerged to help us analyse these huge amounts of data.
Even though we have been using risk analytics for a long time, the new age data science can offer a level of sophistication and benefits that were not possible with traditional methods. Let’s look at some of the areas of risk management where data science is being applied.
Risk Assessment and Intelligence
In a financial institution, large amounts of data is generated in the form of banking transactions, consumer behaviour, market price fluctuations, and economic data. Data analytics can be used to explore and analyze such big data in order to mitigate risks. Since we are dealing with multiple huge data sets, the data science tools can be really helpful in exploring and analyzing these data sets from various perspectives. Such analysis can provide almost real-time intelligence and allow risk managers to detect potential risks and act faster and more effectively in mitigating those risks.
Credit Risk: Predictive Power
Within the credit risk domain, data science can be used to build more robust predictive models to assess loans and borrowers. Apart from the standard datasets such as loan history, banking transaction data, demographics, income, etc, new data from sources such as social media and marketing data can be included in building models to get deeper visibility into customer behaviours. For example, a major life event such as a divorce or a new child, can add significant insights. The traditional data, together with such new information, can help risk managers create highly robust risk indicators. Such big data analytics can also help banks detect early warning signals in their existing loan servicing business. For example, based on their social media postings, can we derive some insight into whether the borrower will continue to service their loan?
Anti-money Laundering
Anti-money laundering and terrorist cases cost a lot to banks as the industry adopts a zero-tolerance approach and faces huge fines for such activities. However, there are serious limitations with the traditional methods to detect and prevent money laundering which are largely rule-based and search-based. For example, the AML systems may be raising an alarm every time it sees a certain keyword. But these methods are not very effective, and are very labour intensive as AML operations staff needs to manually review lots of transactions and reports which is time-consuming and error-prone. With the help of big data and data science, the AML practice can be improved significantly. With the help of statistical analysis and data visualization of both structured and unstructured data, hidden patterns and links can be identified between accounts and transactions, which can then lead to identifying suspicious transactions. Advanced techniques can be used to generate real-time actionable insights and stop money-laundering at its beginning itself.
Financial Market Risks
Data science also has its applications in risk related to financial markets such as counterparty credit risk and market risk. Big data analytics can help in improving the quality and speed of simulation and scenario analysis, such as Monte Carlo simulation (for example, to calculate Credit Valuation Adjustment (CVA) at the portfolio level). These stronger Monte Carlo simulation abilities can help banks price path-dependent derivatives much better than their competitors. Both credit risk and market risk exposures and valuations can be simulated in a much more advanced way.
Operational Risk: Fraud Management
In operational risk, big data and data science can be used to create highly efficient systems to detect and prevent fraudulent activities such as fraudulent/speculative trading, rogue trading, and regulatory breaches. Traditional operational risk management methods are slow and require a case-by-case approach to detect fraud, while traders use newer technologies and strategies to conduct their activities such as chat rooms used in forex frauds. All these data sources can be captured and analyzed along with standard trading and transactional data sources to detect fraud before it becomes a scandal.
While these are some examples of how big data and data science is changing the risk management landscape, there are many other use cases where use of data science can help banks manage risks, improve transparency, reduce costs, and prevent disasters.