How Data Science is Used in Fintech (Financial Technologies)
Financial technology, popularly referred to as FinTech, is one of the fastest growing areas in technology innovation and is a favourite for venture capitalists. Fintech refers to a set of technologies that focus on new ways of delivering banking and financial services to consumers. When you use PayPal, Amazon pay, or your credit card to make a payment online, you the consumer, the ecommerce company, as well as the bank are all using Fintech to make the transaction happen. Over time, Fintech has evolved and disrupted almost all aspects of financial services, including payments, investments, consumer finance, insurance, securities settlement, and cryptocurrencies, among others.
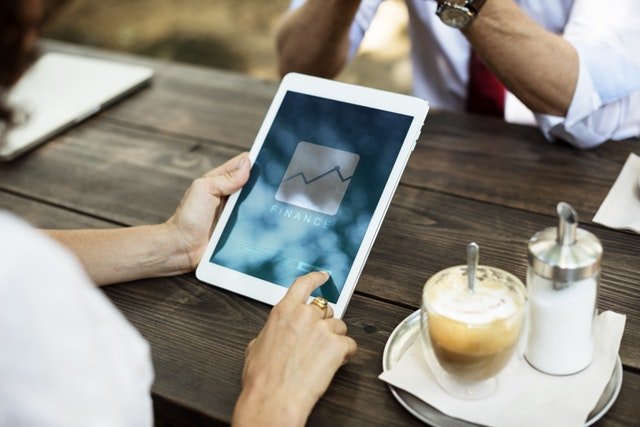
Fintech companies heavily depend on machine learning, artificial intelligence, predictive analytics and data science to simplify financial decision making and provide superior solutions. Let’s take a few examples of fintech and how data science is used in each.
Robo-Advisors
Robo-Advisors are digital platforms that provide algorithm-driven, automated financial planning and investment services to investors. Most of the process is technology driven and the investment decisions are arrived at by algorithms. There is minimum or no human intervention in the entire process. Typically the process starts with collecting information about the client through an online survey where the client’s profile is captured, such as their financial status, risk capacity, future financial goals, etc and then the data is used to processed to provide financial advice or automatically invest client assets in instruments and asset classes best suited for their needs and goals.
Risk Analysis
Credit rating agencies and credit scoring companies like FICO rely on data science and machine learning to provide instant data on borrowers. For example, they use logistic regression to predict the risk of customers and separate good borrowers from bad ones.
Fraud Detection
Data science techniques can be used to identify fraud in financial transactions. Traditionally, identification of fraud has been rule based, and the rules for flagging a transaction had to be set manually. We can now leverage big data and data analytics techniques where vast amounts of online fraudulent transactions can be used and modelled in a way that can help us flag or predict fraud in future transactions. This can be done using data science and machine learning techniques such as Deep Neural Networks (DNNs).
Customer Acquisition and Retention
Banks and financial institutions can use internal and external customer data to create comprehensive customer profiles which could be used to tailor customer experience and provide highly personalised offers. For example, an algorithm could be build to predict what additional products or services the customer would like to purchase based on their historical purchase behaviour. Or for example, what kind of product should be promoted to a certain age group of people.
Insurance Products
Insurance industry is also a big user of data science. Almost every time of insurer uses data science to manage their risk and keep their business profitable. As an example, the claims department in an insurance company uses data science algorithms to separate fraudulent from non-fraudulent transactions. Insurance companies also use data science and big data for other purposes such as credit scoring, customer acquisition, marketing, customer retention, and designing new insurance products.
While these are some examples of how data science can be used in Fintech, there are infact limitless possibilities of its application.